Data Analysis with Machine Learning in Finance: A Comprehensive Guide
PW
Introduction to Data Analysis in Finance
In the fast-paced world of finance, data analysis has become a crucial tool for making informed decisions. With the advent of machine learning, financial analysts can now harness vast amounts of data to uncover patterns, predict market trends, and optimize investment strategies. This comprehensive guide explores how data analysis combined with machine learning is transforming the financial landscape.

The Role of Machine Learning in Finance
Machine learning algorithms have the capability to process and analyze large datasets with precision and speed that far surpasses human capabilities. They help in identifying hidden patterns and correlations that might not be immediately obvious. In finance, this means more accurate predictions of stock prices, better risk management, and enhanced customer service.
Predictive Modeling
Predictive modeling is a cornerstone of machine learning in finance. By analyzing historical data, algorithms can forecast future market movements or credit risks. This empowers financial institutions to make data-driven decisions, reducing potential losses and maximizing returns.

Risk Management
Risk management is another area where machine learning excels. By evaluating historical data and real-time market conditions, algorithms can provide insights into potential risks, allowing companies to devise strategies to mitigate them effectively. This proactive approach can safeguard businesses against unforeseen financial crises.
Data Analysis Techniques
Various techniques are employed in data analysis within finance, each serving a unique purpose. Some of the most common methods include:
- Regression Analysis: Used to forecast changes by understanding relationships between variables.
- Time Series Analysis: Helps in analyzing historical data to predict future trends.
- Clustering: Groups similar data points for better segmentation and analysis.

Integration of Machine Learning
The integration of machine learning into these data analysis techniques enhances their efficacy. For instance, by employing advanced algorithms, financial analysts can achieve a higher degree of accuracy in their models. This leads to more reliable forecasting and better strategic planning.
Challenges and Considerations
While machine learning offers numerous benefits, it is not without challenges. One major issue is the quality of data. Poor quality or incomplete data can lead to inaccurate predictions. Additionally, the complexity of machine learning models requires skilled personnel to interpret results accurately.
Ethical and Regulatory Concerns
The use of machine learning in finance also raises ethical and regulatory concerns. Financial institutions must ensure that their use of data complies with privacy laws and that their algorithms do not perpetuate biases. Transparency in algorithmic decision-making is critical to maintain trust with customers and regulators.
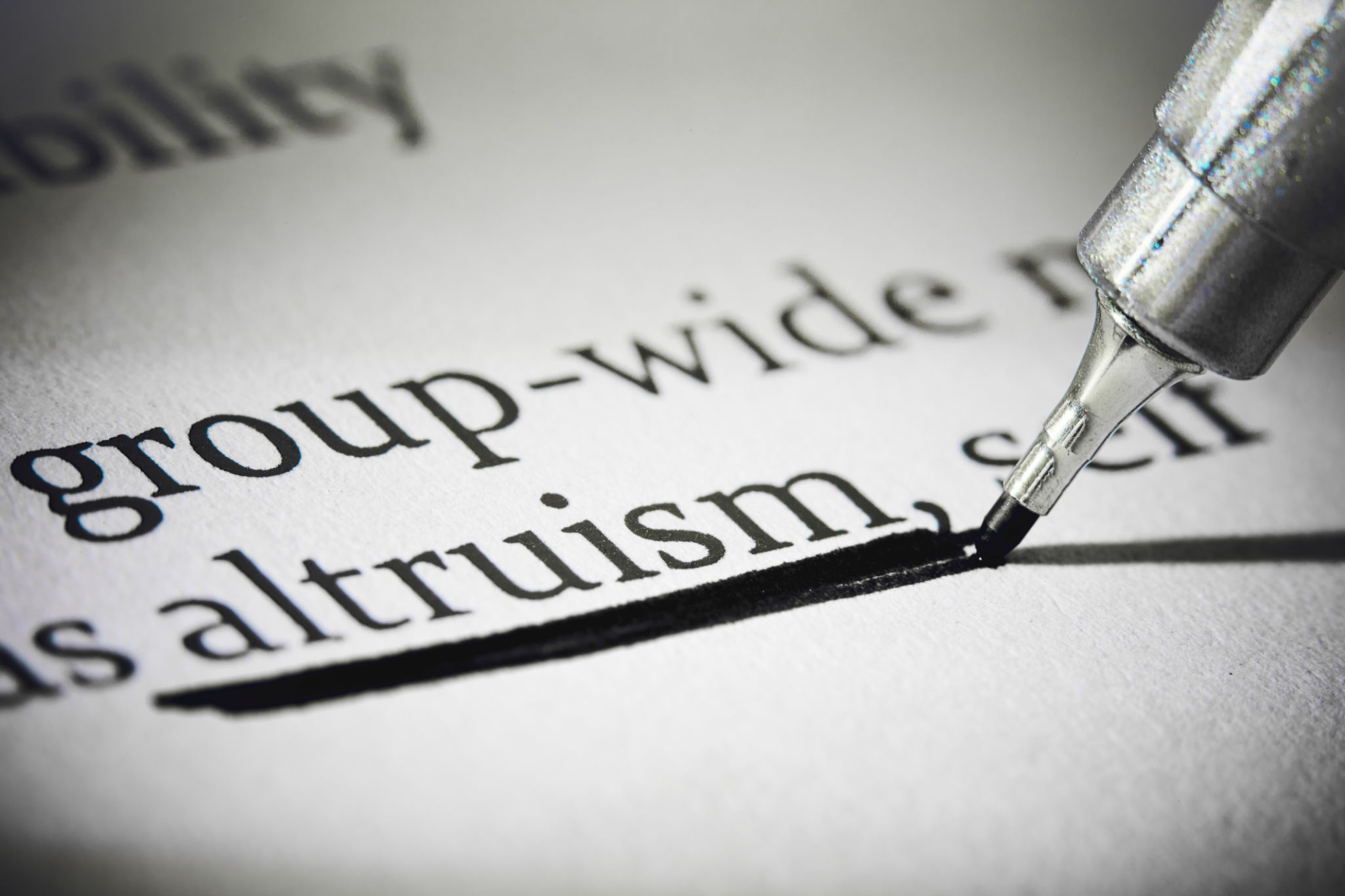
Conclusion
Data analysis with machine learning is revolutionizing the finance sector by providing valuable insights and enhancing decision-making processes. However, as with any powerful tool, it must be used responsibly. By addressing challenges and adhering to ethical guidelines, financial institutions can leverage these technologies to achieve significant competitive advantages.